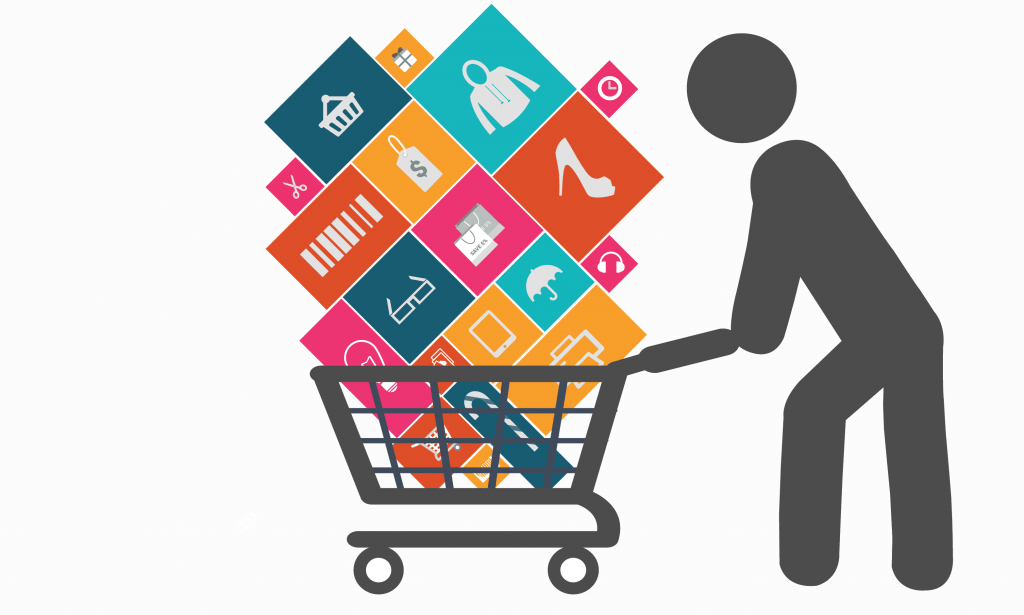
This will be the final chapter covering the ‘fundamentals’ of retail science. We have a foundation established and we will move on to more complicated topics going forward. In the meantime enjoy this entry discussing the intricacies of regular price optimization.
Retail science encompasses five basic areas, regular price, promotion, markdown (clearance), assortment, and replenishment. While all five are exciting areas of research in retail science, I’d like to focus for a few pages on the bedrock of retail, regular-price sales. They can be brick-and-mortar sales or online sales or omnichannel sales bridging online purchase with in-store pickup. The fundamental common thread here is that these are the usual prices for most of the shoppers most of the time.
We’ll explore the individual product and store prices and how they extend to networks of prices and to categories.
The conclusion is that attention to price image is important, but the mathematics of retail science can refine prices to earn an extra percent or two in both revenue and profit that even a good category manager won’t find.
In our college days we sat in a big lecture hall for Economics 101 where a professor put price on one axis and units on another axis and drew a downward-sloping curve with a big “D” for “Demand” next to it. (The “Supply” curve with the big “S” is not interesting here.) The idea is that buyers of some commodity buy more of it when the price is lower and buy less of it when the price is higher. We can delve into the actual mathematics in another place, but I’d like to make a couple of points right here.
What’s important to take away from the Econ 101 classroom is that there is a response to price in product unit sales, fewer units at higher prices. We characterize that response as elasticity, percent change in unit sales per percent change in price. Elasticity less than one, which we call “inelasitic,” has revenue increasing as price increases and elasticity much higher than 2.0, which we call “elastic,” typically has profit decreasing as price increases. (Technically, E=1 is maximum revenue and E=P/(P-C) is maximum profit, where P=price and C=cost.)
Standard retail-science models for the past two decades have modeled elasticity increasing with price, an assumption that promotes mathematical stability along with realistic shopper behavior. As most of our observed elasticities congregate between 1.0 and 1.5, this would suggest there’s more money to be made by raising most of our prices.
RULES
Even if we have a mathematical demand curve, we have business rules that constrain our prices. We have to drive between the lines for our demand models to work.
A product with a competing price isn’t going to follow the same modeled demand above the competitive price as below. The competitive price may be parity (same product, same size) or it may be some equivalized price for a lower or higher brand or a smaller or larger quantity. The competitive pressure is highest when a nearby store or another web page is selling the exact same thing, but even then a retailer can have shopper loyalty that keeps a sale even with an unfavorable price difference.
Maintaining margin is important, selling for enough over cost to make it worthwhile. Even if each product individually isn’t making a profit, we want a community of products (a category perhaps) to be profitable enough to meet business requirements.
Line structure refers to relative pricing of various brands or size or count or dimension. There are dozens, hundred, even thousand of attributes used to compare products and to establish price relationships.
There are also price points (ending in nine) that restrict price selection.
We can design an optimizer to find prices that make the highest demand-driven profit within the rules. It’s a difficult mathematical problem involving some complex algorithms, but we can do it.
BIGGER PICTURE
Let’s go to a bigger picture of retail science. Unit sales are the product of three factors, traffic, share, and count. Traffic is the number of shoppers who enter the store (or web page), share is the fraction who buy a product (web-page conversion rate), and count is how many they buy. We can think of product price as the driver of share and count while a more-global presentation of price drives traffic, getting people into the store (or onto the web page). Reality is a bit more complicated than this simple view, but it gives a good first-order view of retail demand.
There is a concept sometimes called “price image” that expresses the general pricing a store presents to those who shop there, or at least the pricing a category or sub-category presents.
If we use the product-price logic described above on the product prices in a store, we’ll find most of them will be higher than the present prices. The effect of generally-higher prices is fewer shoppers. The traffic picture suggests a retailer wants to maintain a consistent price image for each category or sub-category.
PRICE IMAGE
We can define price image in all kinds of ways using key-value items and other indices of product-price importance, but we won’t be far off if we use the simple metric of overall margin for a category. If a category is priced for a certain margin and we rearrange its product prices to maintain the same margin, the we should have the same price image.
This can be more difficult than it appears. If unit sales are a function of price, then raising or lowering prices can change unit sales enough to cause some unintuitive results. I remember one case of two items, one high-margin and high-elasticity and the other low-margin and low-elasticity, where lowering both prices caused combined margin to increase. Most of the time price behavior isn’t this crazy, but unexpected things can happen in setting retail prices.
So our price-setting dance involves coordinating demand optimization with rules and price image for each category. This is a yet-more-complex calculation we can facilitate by offering the retailer a continuum of choices, a revenue-profit “frontier” of price options for each category.
INDIVIDUAL PRICES
There appears to be a community of retail price managers who feel that satisfying most of the rules and managing price image is all they really need to do. How much wiggle room is there? Doesn’t that pretty-much nail down the prices?
I’ve encountered retailers who really don’t believe in demand elasticity. They believe that price image gets people into their stores and that overall margin makes it profitable to serve those shopper. I remember one fellow gasping in amazement when he dropped a price from six dollars to five and there was a clear jump in unit sales of that product, right there, right away.
Most retailers are a little more savvy that this. They get there is a demand response to price, but figure that the rule constraints and the price image target don’t leave a lot of room for improvement. It’s a push-down-pop-up world out there, push one price down and another price can come up so the end result is about the same, or so some figure.
There’s a level of refinement available to regular-retail-price managers. Within the price-image-constrained pricing of a category, we can push one price down and pop another price up to take advantage of the elasticity imbalances. These push-down-pop-up exchanges of price are hard to find, especially within the business contraints of rules and price-point ending numbers. A computer can use the knowledge we have of elasticity and rules to find the small trade-off price exchanges that allow a retailer to earn an extra one or two percent, sometimes a little more.
There are so many combinations of price-up-price-down changes that a person looking for them would have to search forever while knowing all the individual elasticities. This is where computer optimization isn’t a Nice-To-Have option but is a business requirement, the fine polish on the already-thought-out strategy a retailer worked hard to achieve. We can stay within both rules and price image while finding the small improvements in both revenue and profit.
CONCLUSION
Within the strategic limits of rules and price image, we can use sales history and other statistical tools to estimate individual price-demand elasticity of each product. Once we have each product’s price behavior, we can push some prices up and pull some prices down to get one or two percent more revenue and profit without jeopardizing the traffic flow based on overall price and margin.